This blog post evaluates the performance of new policymaking tools developed in ICT for Governance and Policy Modelling, part of the European Commission’s Seventh Framework Programme (FP7). The author participated in projects co-funded by the programme.
The 21st Century has posed a set of radical new challenges to policymakers. Change comes faster. Problems are less linear and predictable, more systemic and complex. From 9/11 to climate change, from financial crisis to pandemic, governments worldwide are struggling to deal with the so-called “black swans.” As then President of the European Central Bank Jean-Claude Trichet put it in the aftermath of the 2008 financial crisis: “As a policymaker during the crisis, I found the available models of limited help. In fact, I would go further. In the face of the crisis, we felt abandoned by conventional tools.”
To meet these changing demands, researchers developed a set of new instruments particularly suited for a complex, interrelated, nonlinear world. These so-called Policy 2.0 tools brought with them a host of new ideas – and a dog’s breakfast of new jargon to describe them: policy modelling, open government data, visual analytics, collaborate governance, citizen science and behavioural economics. The European Commission, for its part, put real money on the table; The 2009 ICT for Governance and Policy Modelling programme set aside €40 million for research on the most promising new tools. And the author of this blog post led a consortium of researchers behind Towards Policymaking 2.0: The International Research Roadmap for ICT for Governance and Policy Modelling, an article where many of the new techniques were first described and laid out.
Chart 1. New Tools for a New Policy Cycle
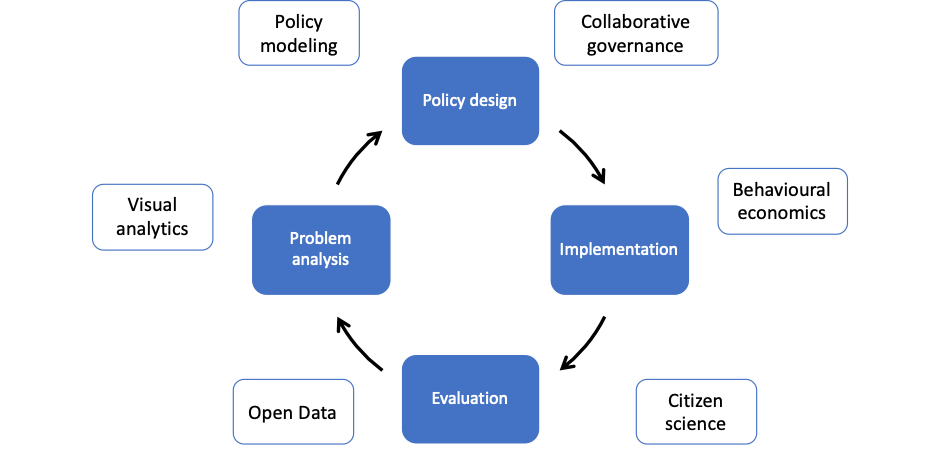
Source: Adapted from Osimo et al., Towards Policymaking 2.0: The International Research Roadmap on ICT for Governance and Policy Modelling (CROSSOVER project, 2013)
But policymaking would be nothing if we didn’t evaluate outcomes and look for evidence. So how, then, did these new tools perform when confronted with the once-in-a-lifetime crisis of a vast global pandemic?
It turns out, some things worked. Others didn’t. And the question of how these new policymaking tools functioned in the heat of battle is already generating valuable ammunition for future crises.
So what worked?
Policy modelling – an analytical framework designed to anticipate the impact of decisions by simulating the interaction of multiple agents in a system rather than just the independent actions of atomised and rational humans – took centre stage in the pandemic and emerged with reinforced importance in policymaking. Notably, it helped governments predict how and when to introduce lockdowns or open up. But even there uptake was limited. A recent survey showed that of the 28 models used in different countries to fight the pandemic were traditional, and not the modern “agent-based models” or “system dynamics” supposed to deal best with uncertainty. Meanwhile, the concepts of system science was becoming prominent and widely communicated. It became quickly clear in the course of the crisis that social distancing was more a method to reduce the systemic pressure on the health services than a way to avoid individual contagion (the so called “flatten the curve” project).
Open government data has long promised to allow citizens and businesses to build new services at scale and make government accountable. The pandemic largely confirmed how important this data could be to allow citizens to analyse things independently. Hundreds of analysts from all walks of life and disciplines used social media to discuss their analysis and predictions, many becoming household names and go-to people in countries and regions. Yes, this led to noise and a so-called “infodemic,” but overall it served as a fundamental tool to increase confidence and consensus behind the policy measures and to make governments accountable for their actions. For instance, one Catalan analyst demonstrated that vaccines were not provided during weekends and forced the government to change its stance. Yet it is also clear that not all went well, most notably on the supply side. Governments published data of low quality, either in PDF, with delays or with missing data due to spreadsheet abuse.
In most cases, there was little demand for sophisticated data publishing solutions such as “linked” or “FAIR” data, although particularly significant was the uptake of these kinds of solutions when it came time to share crucial research data. Experts argue that the trend towards open science has accelerated dramatically and irreversibly in the last year, as shown by the portal https://www.covid19dataportal.org/ which allowed sharing of high quality data for scientific research.
Visual analytics promised to address multidisciplinary challenges and to improve understanding of complex policy issues by placing visualisation not at the end of the process but as part of the analytical work. Building on open data, visual analytics methods experienced a dramatic surge in popularity. Not only visualisations were everywhere, but they were widely discussed. And because of the heavy polarisation of the debate, particularly popular were tools that enable users to choose between different visualisations – for example in the argument whether absolute or relative value (weighted on population) should be considered to assess the performance of countries – Financial Times Coronavirus Tracker being probably the go-to visualisation. While this sometimes became a sort of childish competition between countries, it undeniably allowed society to widen the awareness of data analytics, for instance by popularising the difference between linear and logarithmic charts.
But other new policy tools proved less easy to use and ultimately ineffective. Collaborative governance, for one, promised to leverage the knowledge of thousands of citizens to improve public policies and services. In practice, methodologies aiming at involving citizens in decision making and service design were of little use. Decisions related to lockdown and opening up were taken in closed committees in top down mode. Individual exceptions certainly exist: Milan, one of the cities worst hit by the pandemic, launched a co-created strategy for opening up after the lockdown, receiving almost 3000 contributions to the consultation. But overall, such initiatives had limited impact and visibility. With regard to co-design of public services, in times of emergency there was no time for prototyping or focus groups. Services such as emergency financial relief had to be launched in a hurry and “just work.”
On the other hand, precisely for this reason, one could see the benefits of having invested in good service design with a long-term perspective: composable, usable, interoperable services that could be set up quickly, webpage templates that delivered clear consistent messages across different pages, and most of all an agile team able to react quickly and effectively. This “silent” service design was instrumental in helping set up quickly emergency relief for the self-employed in Germany and communication campaigns in the UK government website. In other words, unusable interfaces, data silos, complex processes and bureaucratic jargon became unsurmountable obstacles for citizens at a time of emergency, when digital was the only channel.
Citizen science promised to make every citizen a consensual data source for monitoring complex phenomena in real time through apps and Internet-of-Things sensors. In the pandemic, there were initially great expectations on digital contact tracing apps to allow for real time monitoring of contagions, most notably through bluetooth connections in the phone. However, they were mostly a disappointment. Citizens were reluctant to install them. And contact tracing soon appeared to be much more complicated – and human intensive – than originally thought. The huge debate between technology and privacy was followed by very limited impact. Much ado about nothing.
Behavioural economics (commonly known as nudge theory) is probably the most visible failure of the pandemic. It promised to move beyond traditional carrots (public funding) and sticks (regulation) in delivering policy objectives by adopting an experimental method to influence or “nudge” human behaviour towards desired outcomes. The reality is that soft nudges proved an ineffective alternative to hard lockdown choices. What makes it uniquely negative is that such methods took centre stage in the initial phase of the pandemic and particularly informed the United Kingdom’s lax approach in the first months on the basis of a hypothetical and unproven “behavioural fatigue.” This attracted heavy criticism towards the excessive reliance on nudges by the United Kingdom government, a legacy of Prime Minister David Cameron’s administration. The origin of such criticisms seems to lie not in the method shortcomings per se, which enjoyed success previously on more specific cases, but in the backlash from excessive expectations and promises, epitomised in the quote of a prominent behavioural economist: “It’s no longer a matter of supposition as it was in 2010 […] we can now say with a high degree of confidence these models give you best policy.”
Three factors emerge as the key determinants behind success and failure: maturity, institutions and leadership.
First, it is clear that many advanced Policy 2.0 tools turned out not to be mature enough to deal with a crisis so extreme – only the most mature of them worked. But this should not be perceived as a clear-cut judgment that such methods are ineffective. Using a metaphor from the coronavirus, deploying such tools during a pandemic is like vaccinating everyone before phase three clinical trials. Experimentation in public policy is much slower than in natural science, and there is a big difference between pilots and full-scale deployment – particularly in the midst of a pandemic. What we need is more experimentation and better data – and a more honest evaluation of the performance.
Second, sophisticated tools are no substitute for functional institutions, well-designed processes and high-quality reference data. When it came to dealing with the pandemic, countries with efficient health systems, trusted public services and competent decision-makers were able to alleviate the problem or deal better with the consequences. Software can help, but only when accompanied by sufficiently good hardware and infrastructure. Models are effective, but only if fed by high-quality data. Sophisticated simulations cannot make up for the lack of investment in primary care.
But most of all, the lesson is that challenges of such a magnitude can’t be solved by relying on a silver bullet. They can only be solved by trying out and balancing different solutions. More than ever, policymakers need to lead, not simply to rely on trusted advisors: they should be informed and capable of managing different tools and different expertise. They need to be qualified enough to understand the assets and limitations of different methods. Indeed, the common factor behind the failure stories of the pandemic is the over-reliance on one single model, or policy tool, or scientific theory, or highly rated individual. To give a concrete example, bold policy decisions such as herd immunity cannot be based on unproven theoretical statements, excessive trust in individual experts or models based on limited data. More than the inherent properties of each tool, what matters is the institutional capacity to use them properly and proportionately to the available evidence.
David Osimo is director of research at the Lisbon Council.
Download in PDF